Data Analysis and Analytics
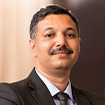
While “Data” as a standalone term doesn’t attract much interest, the topic “Data Analysis and Analytics” is appealing because of the terms Analysis and Analytics.
An elementary human behavior works behind this: the desire to cherish the outcome without focusing on the constituents that make it happen. For instance, we all like desserts after food and appreciate the final output, which is dessert. But anyone hardly ever wonders what goes behind cultivating the resin, which is an ingredient of that dessert, what goes behind transporting and preserving the resin, and what the dessert preparation process is. The critical thing to understand is that if the data on the above aspects are unavailable, neither analysis nor analytics on the subject can happen.
Since we mentioned analysis and analytics, let’s touch upon the basic understanding of these terms. We all know the DIKW (Data, Information, Knowledge, Wisdom) pyramid, often used to illustrate that data leads to information, information to knowledge, and knowledge to wisdom. In layperson’s terms, knowledge is on a subject that has happened in the past, which is what analysis is. And wisdom is the application of knowledge on a topic that is yet to happen: this is called analytics. Data Analysis is the process of understanding what has happened so far basis the data. In contrast, Data Analytics is the process of application of data analysis into data models, which helps predict outcomes with the changes in coefficients and variables of data models.
Before we go any further on Analysis and Analytics, let us acknowledge that the basis for everything is Data. If Data is missing, we lose all paths to information, knowledge, and wisdom.
Understanding DIKW Pyramid
While many scholars have elaborated on the subject, a few referred to below are the ones I find easy to understand and relevant to the current discussion topic.
Data – Jennifer Rowley categorizes data “as being discrete, objective facts or observations, which are unorganized and unprocessed and therefore have no meaning or value because of lack of context and interpretation.”
Information – While Data is referred to as unorganized and unprocessed facts or observations, structuring the same data in a relevant manner makes it information. Again, Rowley describes information as “organized or structured data, which has been processed in such a way that the information now has relevance for a specific purpose or context, and is therefore meaningful, valuable, useful and relevant.”
For example, if there is a raw dump of facts around the source, destination, departure time, arrival time, etc., of the public transport system you use for transit to and from your workplace, it’s just Data. But if the same data is presented in a structured manner which you can consume as a customer, it’s information.
Knowledge – We now understand that information is a structured representation of a set of facts in a context, making it relevant for consumption. Assimilation of a collection of data over a frequency of occurrences, along with capturing variations in the process and outcome, with appropriate reasoning of the causes of deviations and collated in the way it can be consumed to provide answers to 5W1H, is knowledge.
Let’s decode this a bit. The term “frequency of occurrences” signifies that something happens continuously over time. The “set of information” denotes all occurrences’ structured data. The “causes of variations” indicate variations in outcomes over the happenings, and analysis (subjective and objective) is done to understand the same. A simple example of knowledge is a performance report of any process over a while.
The relevance of “Analysis” in the context of knowledge is significant. Unless an analysis is performed on the information set, learning on the subject cannot be attained. As mentioned, analysis is based on information gathered from occurrences that have already happened.
Wisdom – We had the data; the data was put in a structure with a context to provide information, and a set of information was collated over a period and analyzed to arrive at the knowledge. Now what! What do we do with this knowledge? While knowledge is excellent for understanding what has happened, how can we apply the knowledge for a desired outcome tomorrow?
And this is where Analytics comes into play. Wisdom is the application of derived knowledge in a manner where the outcomes can be predicted based on the variations in influencing factors. The required controls on influencing factors can be determined to deliver the desired result. Analytics is the process of identifying the data model with variables as the influencing factors and hence predicting the outcome basis the changes in the coefficients of the variables.
Please note that the explanation of DIKW above is done in the context of data, not in literal terms where knowledge and wisdom have extremely subjective connotations.
Back to Data
As mentioned multiple times above, Data is the key. The emphasis on data is being made repeatedly because this is the aspect most organizations struggle with. Great tools are also available to arrive at Information, Knowledge, and Wisdom if data is available. But in most cases, the challenge is the need for more data.
“No, I disagree. I deal with data on a minute-to-minute basis, and if you ask me for any data, I will provide it.” Absolutely, and that is what the issue is. Data is lying across the organization fragmentedly at various sources in various formats. Any information needed is a new task of gathering all data from all sources and providing it in a structured manner, again for one-time use only. And this tomorrow again becomes another data without any relevance for anyone else. Hence, the need today is to have a system that captures or maintains all data in a data repository to generate required information, knowledge, or wisdom at any time.
While there are various methods to arrive at the set of data that needs to be collated, a simple way to start on the journey is to document all business and operational processes, list down the KPIs for each of the processes, and begin capturing KPIs, measurement parameters of the KPIs, and the influencing factors for the same. This will serve as a great starting point.
Remember, structuring data with a context is information. And if all the data is available in a reusable manner, information can be provided with ease in abundance. And indeed, the journey to knowledge and wisdom also becomes smoother.
Let’s understand the overall lifecycle of data to wisdom with an example we deal with daily, the Incident Management Process. Information Technology Infrastructure Library (ITIL) defines Incident Management as Service Management practice for managing service interruptions. As discussed above, listed are the KPIs, the Measurement Parameters for the KPIs, and the influencing factors. The unfolding of the same will be as below.
While the above is perfectly correct, typically discussed during performance reviews for the process, we often see an opportunity to improve how we deal with data, information, and knowledge in such cases. For example,
- The information and knowledge on process performance are used retrospectively to understand what impacted the performance and what we intend to have for enhanced controls. Control on the outcome can be further enhanced by having a method to collate data over an extended period and presenting the outcome as an equation/data model with factors influencing SLA as the variables.
- Information on process performance gets consumed discretely for a period of execution. Having the above method enhanced the possibility of comparing the performance over some time and also in predicting the performance for the future.
- The most important aspect is to expand the possibility of collating more factors that can influence performance. For example, Resolution SLA may have correlations with the tickets per device ratio, the ratio of different levels of engineers in the team, the number of Knowledge Articles available, the number of new provisioning done over the duration, the ratio of tickets over different shifts of the day, etc.
Hence, expanding the horizon of data being collated continuously is always suggested. It can then be used in a structured manner as information and can also be used for knowledge and wisdom with the help of appropriate Analysis and Analytics.